AI and Neural Networks: Bridging the Gap Between Artificial and Biological Intelligence
Explore how AI and neural networks bridge the gap between artificial and biological intelligence by simulating cognitive processes and enhancing our understanding of brain function. Learn about innovative developments, real-world applications, and future research in AI-driven neural networks.
The fusion of Artificial Intelligence (AI) and neural networks is bridging the gap between artificial and biological intelligence, simulating cognitive processes and enhancing our understanding of brain function. This post delves into the advancements in AI-driven neural networks, exploring how they mimic the human brain's structure and function. By examining innovative developments, real-world applications, and future research directions, we highlight the transformative potential of AI in neuroscience. Discover how AI and neural networks are revolutionizing the field, offering new insights into brain function and paving the way for future innovations in cognitive neuroscience and artificial intelligence.
The fusion of Artificial Intelligence (AI) and neural networks is bridging the gap between artificial and biological intelligence, revolutionizing cognitive neuroscience by simulating cognitive processes and enhancing our understanding of brain function. This post delves into the advancements in AI-driven neural networks, exploring their transformative potential in neuroscience.
Neural networks are computational models designed to mimic the neural architecture of the brain, consisting of interconnected nodes, or neurons, that process and transmit information. These models, particularly deep learning algorithms, have revolutionized AI by enabling systems to learn from vast amounts of data, identify patterns, and make predictions. By simulating neural processes, AI-driven neural networks offer a powerful tool for understanding cognitive functions and brain activity.
The integration of AI and neural networks has led to several key developments. Deep learning algorithms, such as convolutional neural networks (CNNs) and recurrent neural networks (RNNs), enable AI to process complex data, recognize patterns, and simulate cognitive functions with high accuracy. In neuroscientific applications, AI-driven neural networks model brain activity, study neural mechanisms underlying cognition, and predict neural responses to stimuli. These models help researchers understand brain function and develop interventions for neurological disorders. Additionally, neural networks simulate specific brain functions, such as vision and language processing, providing insights into how these functions emerge from neural activity.
Real-world applications of AI-driven neural networks include medical diagnostics, where AI analyzes medical imaging data to detect and diagnose neurological disorders like Alzheimer's and epilepsy. By identifying patterns in brain scans, these models offer accurate and early diagnosis, improving patient outcomes. Neural networks enhance Brain-Computer Interfaces (BCIs) by interpreting neural signals and predicting user intentions, allowing individuals with disabilities to control external devices using their thoughts, enhancing communication and mobility. AI-driven neural networks also develop cognitive enhancement technologies that improve memory, attention, and problem-solving skills, offering new opportunities for education and mental health interventions.
However, several challenges and limitations must be addressed. The complexity of neural networks makes it challenging to interpret their functioning and understand how they make decisions. Ensuring transparency and interpretability is crucial for trust and ethical AI use. Training neural networks requires vast amounts of high-quality data, which is time-consuming and expensive to collect and curate. The use of neural networks in neuroscience raises ethical issues related to data privacy, consent, and the potential for misuse. Addressing these concerns is essential for responsible AI development.
Future research in AI-driven neural networks focuses on advancing algorithms to improve accuracy, efficiency, and interpretability. Researchers are exploring novel models, such as generative adversarial networks (GANs) and reinforcement learning, to enhance AI capabilities. Efforts to enhance data efficiency through techniques like transfer learning and unsupervised learning aim to make neural networks more accessible and applicable to various fields. Developing ethical guidelines and frameworks is crucial to ensure the responsible use of neural networks in neuroscience, with engagement from stakeholders and the public to address ethical concerns and build trust.
AI-driven neural networks are transforming cognitive neuroscience by simulating cognitive processes and enhancing our understanding of brain function. By bridging the gap between artificial and biological intelligence, these models offer powerful tools for studying brain activity and developing innovative AI applications. Future research should focus on advancing algorithms, enhancing data efficiency, and addressing ethical concerns to unlock the full potential of AI-driven neural networks responsibly and ethically.
Please log in to read the full post and explore these exciting developments in detail.
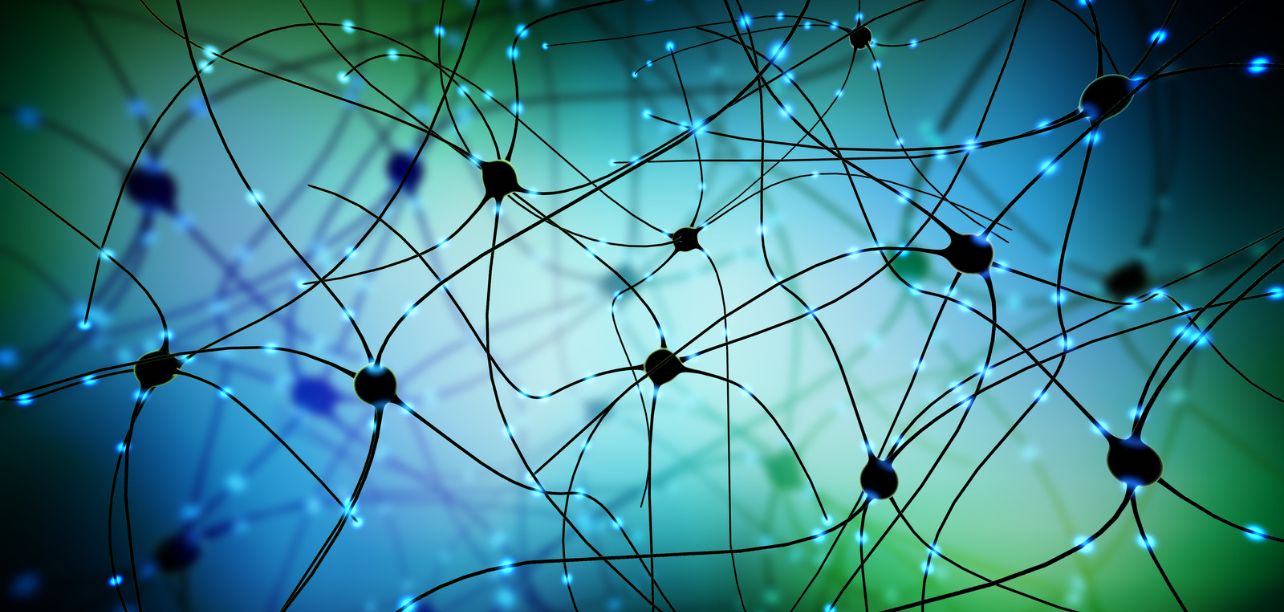