Neural basis of computational neuroscience
Explore the neural basis of computational neuroscience, from its background to potential applications, challenges and limitations. Learn about neural networks, synaptic plasticity and brain-computer interfaces and discover the impact of computational neuroscience on healthcare, education, robotics, gaming, and marketing. Additionally, we discuss the ethical and privacy concerns, complexity, non-linearity, and reproducibility challenges associated with this field.
Computational neuroscience is a field that involves using mathematical modeling and theoretical approaches to study the complex relationships between brain and behavior. It seeks to understand the brains information processing abilities and how they emerge from the interplay of neural systems. In this post, we will delve into the neural basis of computational neuroscience and explore its potential applications, challenges, and limitations.
Computational neuroscience is an interdisciplinary field that draws on expertise from various disciplines, including neuroscience, physics, mathematics, and computer science. It seeks to understand the neural basis of behavior and cognition by developing mathematical models and computer simulations of the brains activity.
One of the most powerful tools used in computational neuroscience is the artificial neural network, which is a mathematical model of the way real neurons interact to process information. Neural networks are used to perform tasks like image recognition, natural language processing, and speech recognition, and their goal is to create machines that can learn from experience. Synaptic plasticity, the ability of synapses to change their strength based on the activity of the neurons involved, is another essential concept in computational neuroscience. It plays a crucial role in learning and memory and is a crucial area for developing effective therapies for conditions like Alzheimers disease and other memory disorders.
One of the most exciting potential applications of computational neuroscience is in healthcare. By understanding the neural basis of disease and dysfunction, computational neuroscience can inform the development of new treatments and interventions for neurological and psychiatric disorders. For example, the study of neural prosthetics and brain-computer interfaces could lead to new ways to treat paralysis, while the study of deep learning and imaging could lead to new ways to diagnose and treat mental health disorders like depression and anxiety.
Another potential application of computational neuroscience is in education. By providing insights into how people learn and remember information, computational neuroscience can inform the development of more effective teaching methods and educational technologies. For example, the study of neural plasticity could lead to new ways of training the brain to improve learning and memory.
Robotics and AI are two more fields that could see significant impacts from computational neuroscience. By mimicking the structure and function of the brain, researchers are developing new approaches to machine learning and artificial intelligence. This could have implications for fields that rely on robotics and automation, including manufacturing, transportation, and healthcare.
Gaming and entertainment are two additional areas that could see significant benefits from computational neuroscience. The study of virtual reality and gaming has the potential to enhance the gaming and entertainment industry by developing more immersive and engaging experiences. Additionally, the insights gained from neuro-marketing research - a new emerging area - can optimize marketing campaigns to create more appealing products to consumers.
However, there are also significant challenges and limitations facing computational neuroscience. One of the biggest challenges is the lack of large-scale, reliable datasets required to develop accurate models of neural activity. The human brain is incredibly complex, and there is still much work to be done to understand the detailed structure of the brains neural circuits, the interaction between different neurons and their inputs, and its non-linearity, which is a challenging computational problem. As such, more accessible and high-resolution imaging techniques and computational methods are essential, and collaboration between experts in neuroscience, computer science, physics, and mathematics is necessary.
Another significant challenge is the ethical and privacy concerns raised by emerging technology like brain-computer interfaces and neuro-marketing, which could interfere with individuals privacy. Additionally, computational neuroscience research face reproducibility problems that threaten researchs scalability and reliability. Thus, new standards for version control, data, and sharing code must be developed and followed consistently.
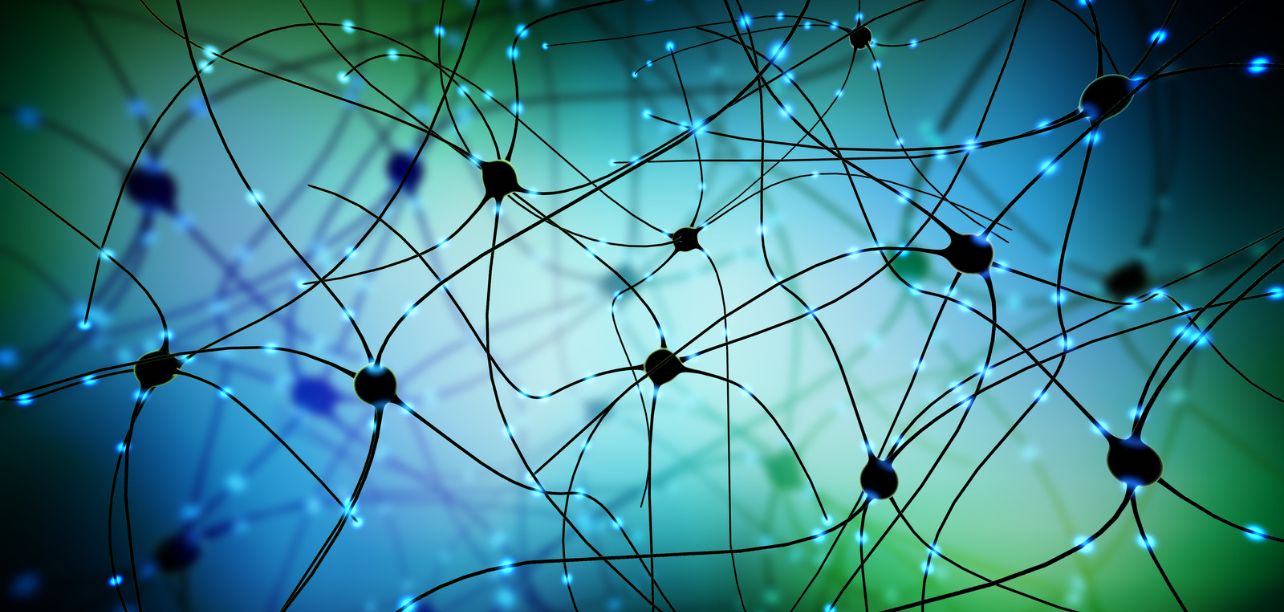